In one of the previous blogs, we described neurons and how they generate electrical spikes. Here, we’ll consider the fact that a neuron is never alone, and it exists in a network. This network can be reorganized via the process of neuroplasticity which leads to changes in neuronal signalling.
Neuroplasticity is the ability of the brain to reorganize its connections. This can involve creating new neurons (neurogenesis), new synapses (synaptogenesis), or modulating the existing synaptic connections (by making them stronger or weaker). The latter includes complex biochemical changes in the existing synapses.
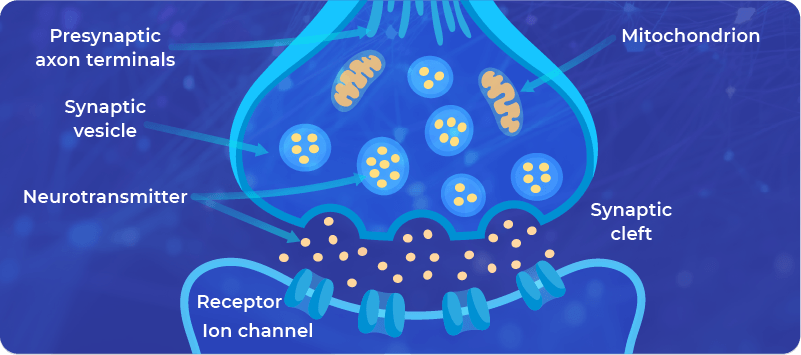
The modulation of existing synapses includes the changes in (i) the structure of the synapses, and (ii) the signaling pathways. Here’s a brief description of both:
1. The changes in the structure of the synapses:
Synapses are built from membranes which contain:
- Channels allowing the controlled transport of molecules through them, for example, Calcium, and
- Receptors allowing the triggering of a signaling pathway involved in the signal transmission.
2. The changes in the signaling pathways ensure the communication in the synapses, for example, the amount of neurotransmitter that is available for processing of the signal.
What triggers neuroplasticity?
Neuroplasticity in the living brain can be triggered by many factors, practically by any sensory stimuli that reach our brain.
This is particularly true when we do something new, such as studying, reading, solving problems, learning new movements, or any new experiences – both in the external and internal world (through emotions, by which we sense our internal signals).
Neuroplasticity can also occur in vitro, not only in the living brain.
We can induce neuroplasticity in vitro, on brain slices, organoids, or cell cultures via chemical or electrical stimulation.
In vitro, we have only nervous cells, sometimes accompanied by glial cells, but we do not have whole organisms attached to them, which can communicate with neurons through sensory stimuli. Therefore, we can stimulate neurons directly with chemical and electrical signals.
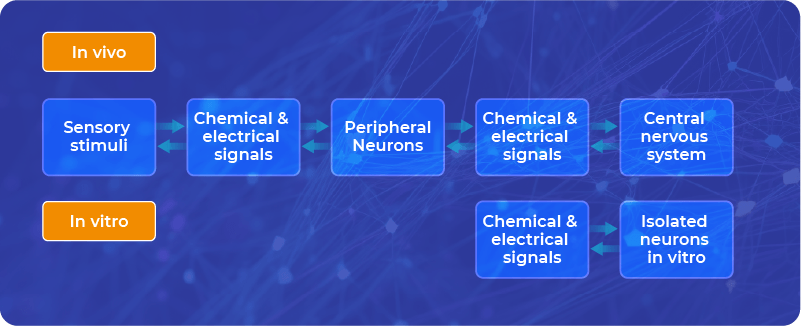
Brain slices vs. single neurons
Brain slices are obtained by cutting the brain sample into tiny sections (‘slices’), each containing thousands of neurons.
In vitro (more precisely ex vivo in this context), we can observe how these fragments of living tissue behave in certain controlled situations. There are many studies on brain slices that are derived from animal brains – to show the mechanisms of neuronal signaling, including neuroplasticity.
On the other hand, there are also precise studies on single neurons with patch clamping or even more rigorous, fully in silico models of neurons, where all the conditions are controllable.
What we do at FinalSpark is something in between brain slices and single neurons.
FinalSpark tries to harness the natural ability of neurons to quantify, store, and process information.
To keep it simple, in the following we focus on a narrow space of neuroplasticity, triggered by electrical stimulation.
At FinalSpark, we use neurospheres, which are round structures consisting of around 10’000 neurons each. These round structures are connected to 8 electrodes, which are used for bidirectional communication of the electrical signal as described before .
In short, we send electrical signals using a digital-to-analog converter (DAC) and we collect the signal from neurons via an analog-to-digital converter (ADC).
Each electrode is only connected to a few neurons; therefore, we have limited information about the whole neurosphere. Our objective is not to figure out the structure of each neuronal connection but rather to trigger functional changes in the electrical behavior of the neurosphere.
How can we perform functional changes in the behavior of the neurons in vitro?
The Hebbian rule states that the ‘neurons which fire together, wire together’. Hebb assumed that the strength of connections is adjusted via spike-timing-dependent plasticity. This means that neuroplasticity is affected both by time and location of the spikes.
Specifically, to strengthen the connection between two neurons, it’s needed that the spike occurs first as an input spike and is followed by an output spike from the same neuron (coincidence detection).
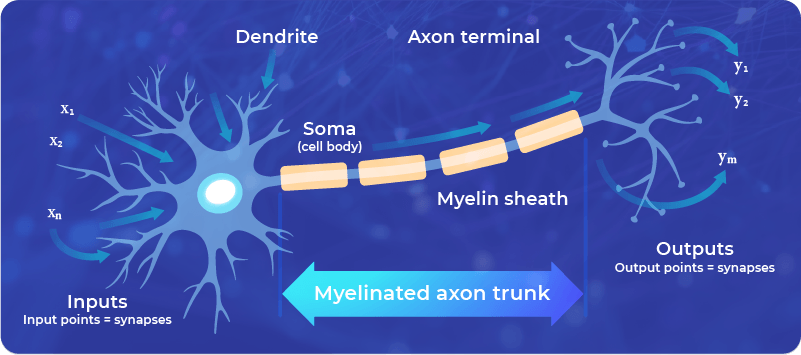
However, this is a simplified approach that is often used in mathematical models, such as neural networks. For real living neurons, the Hebb rule doesn’t cover all the possible behavior of the neuronal signaling.
Also, as a mathematical model, it has limitations, since it is unstable, which means that continuous stimulation, according to the Hebb rule, would lead to an exponential unlimited increase of the synaptic connection – which is impossible in practice.
The Hebb rule, however, helped inspire the design of some computational neural networks, which follow the principles learned from neuroscience. It also helps us to understand the general principle of neuroplasticity: Neuronal connections are strengthened by using them (the more the connection is used, the more it is reinforced).
Artificial models vs. real neural tissue.
Of course, all mathematical models are always a simplified version of reality, which helps to grasp the general principles and test simple hypotheses. But a real living neuron is much more complex in structure and functionality than any artificial model existing.
Data obtained from the artificial models can be used to guide our approach to the stimulation of real neurons in vitro, as these simplified artificial models are also easier to analyze and understand.
The objective of FinalSpark is to modify the living tissue in a predictable, controlled way, which would allow performing computations. We believe that this is the future of the emerging field of biocomputing, in which biological elements are used as hardware. After all, what biological matter can be better for computations than a living neuron?